Leveraging Energy Consumption Patterns for Cyberattack Detection in IoT Systems
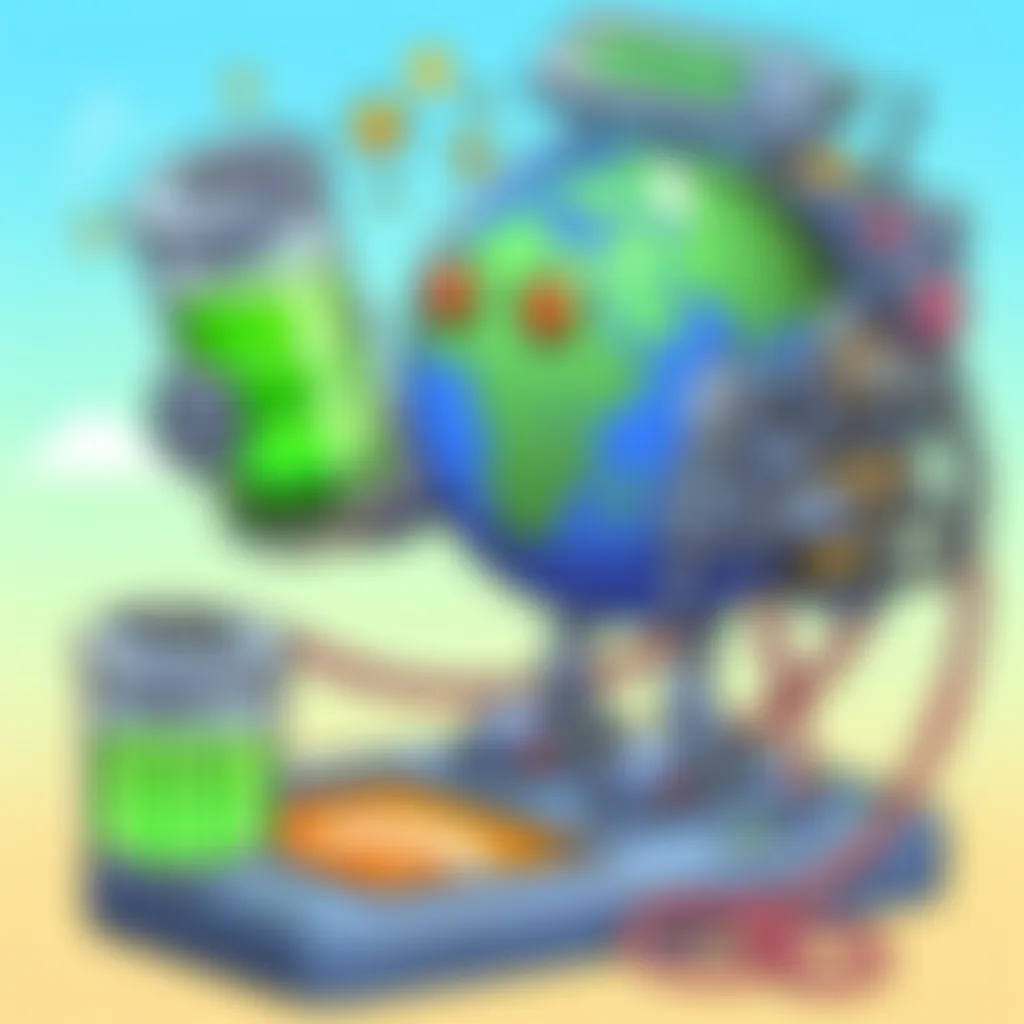
Leveraging Energy Consumption Patterns for Cyberattack Detection in IoT Systems [announcement]
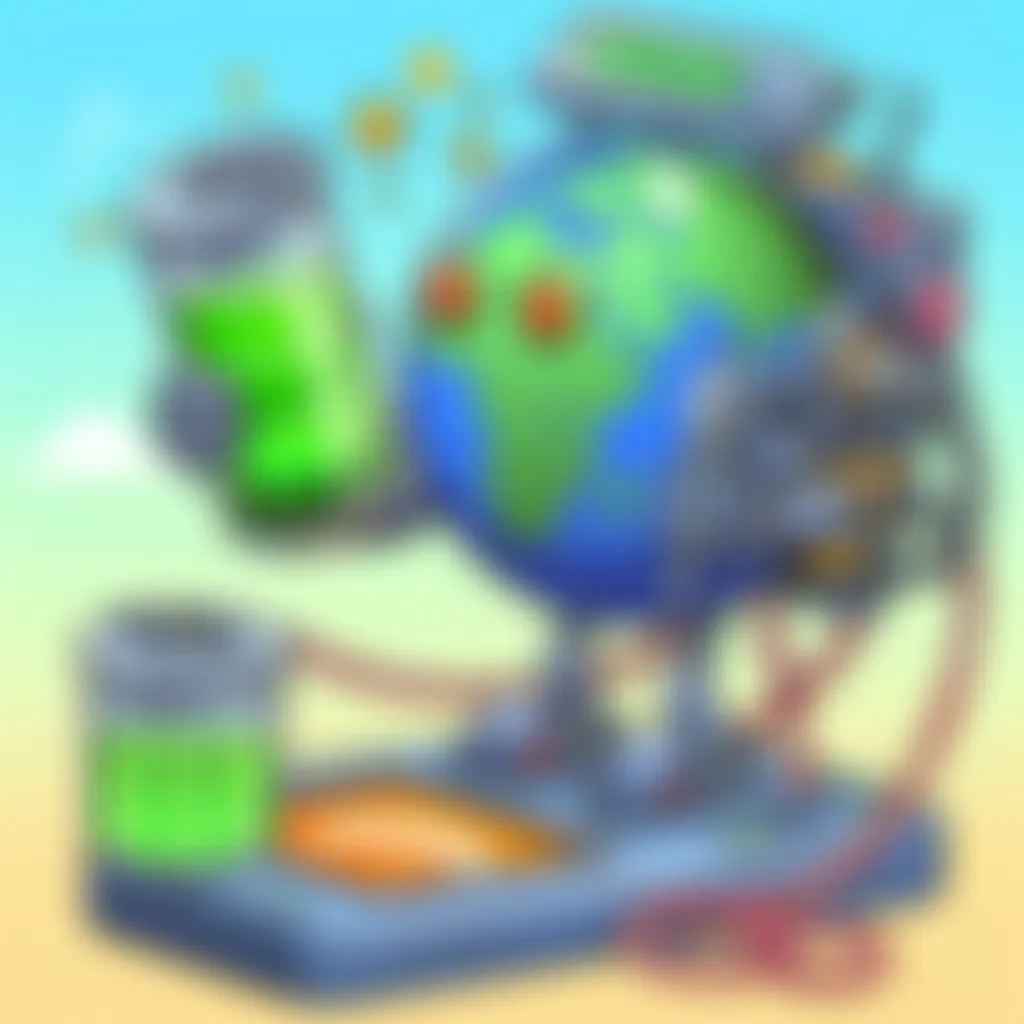
This document provides a comprehensive analysis of the energy consumption of smart devices during cyberattacks, focusing on various aspects critical to understanding and mitigating these threats: types of cyberattacks, detection techniques, benefits and drawbacks, applicability across industries, integration options.
This qualitative analysis provides valuable insights for cybersecurity professionals, IoT specialists, and industry stakeholders. The analysis is beneficial for enhancing the security and resilience of IoT systems, ensuring the longevity and performance of smart devices, and addressing the economic and environmental implications of increased energy consumption during cyberattacks. By leveraging advanced detection techniques and integrating them with existing security measures, organizations can better protect their IoT infrastructure from evolving cyber threats.
----
The proliferation of smart devices and the Internet of Things (IoT) has revolutionized various aspects of modern life, from home automation to industrial control systems. However, this technological advancement has also introduced new challenges, particularly in the realm of cybersecurity. One critical area of concern is the energy consumption of smart devices during cyberattacks, which can have far-reaching implications for device performance, longevity, and overall system resilience.
Cyberattacks on IoT devices (DDoS attacks, malware infections, botnets, ransomware, false data injection, energy consumption attacks, and cryptomining attacks) can significantly impact the energy consumption patterns of compromised devices, leading to abnormal spikes, deviations, or excessive power usage.
Monitoring and analyzing energy consumption data has emerged as a promising approach for detecting and mitigating these cyberattacks. By establishing baselines for normal energy usage patterns and employing anomaly detection techniques, deviations from expected behavior can be identified, potentially indicating the presence of malicious activities. Machine learning algorithms have demonstrated remarkable capabilities in detecting anomalies and classifying attack types based on energy consumption footprints.
The importance of addressing energy consumption during cyberattacks is multifaceted. Firstly, it enables early detection and response to potential threats, mitigating the impact of attacks and ensuring the continued functionality of critical systems. Secondly, it contributes to the overall longevity and performance of IoT devices, as excessive energy consumption can lead to overheating, reduced operational efficiency, and shortened device lifespan. Thirdly, it has economic and environmental implications, as increased energy consumption translates to higher operational costs and potentially greater carbon emissions, particularly in large-scale IoT deployments.
Furthermore, the integration of IoT devices into critical infrastructure, such as smart grids, industrial control systems, and healthcare systems, heightens the importance of addressing energy consumption during cyberattacks. Compromised devices in these environments can disrupt the balance and operation of entire systems, leading to inefficiencies, potential service disruptions, and even safety concerns.
ENERGY CONSUMPTION IMPLICATIONS
📌 Detection and Response to Cyberattacks: Monitoring the energy consumption patterns of IoT devices can serve as an effective method for detecting cyberattacks. Abnormal energy usage can indicate the presence of malicious activities, such as Distributed Denial of Service (DDoS) attacks, which can overload devices and networks, leading to increased energy consumption. By analyzing energy consumption footprints, it is possible to detect and respond to cyberattacks with high efficiency, potentially at levels of about 99,88% for detection and about 99,66% for localizing malicious software on IoT devices.
📌 Impact on Device Performance and Longevity: Cyberattacks can significantly increase the energy consumption of smart devices, which can, in turn, affect their performance and longevity. For instance, excessive energy usage can lead to overheating, reduced operational efficiency, and in the long term, can shorten the lifespan of the device. This is particularly concerning for devices that are part of critical infrastructure or those that perform essential services.
📌 Impact of Vulnerabilities: The consequences of IoT vulnerabilities are far-reaching, affecting both individual users and organizations. Cyberattacks on IoT devices can lead to privacy breaches, financial losses, and operational disruptions. For instance, the Mirai botnet attack in 2016 demonstrated the potential scale and impact of IoT-based DDoS attacks, which disrupted major online services by exploiting insecure IoT devices.
📌 Economic and Environmental Implications: The increased energy consumption of smart devices during cyberattacks has both economic and environmental implications. Economically, it can lead to higher operational costs for businesses and consumers due to increased electricity bills. Environmentally, excessive energy consumption contributes to higher carbon emissions, especially if the energy is sourced from non-renewable resources. This aspect is crucial in the context of global efforts to reduce carbon footprints and combat climate change.
📌 Energy Efficiency Challenges: Despite the benefits, smart homes face significant challenges in terms of energy efficiency. The continuous operation and connectivity of smart devices can lead to high energy consumption. To address this, IoT provides tools for better energy management, such as smart thermostats, lighting systems, and energy-efficient appliances. These tools optimize energy usage based on occupancy, weather conditions, and user preferences, significantly reducing energy waste and lowering energy bills.
📌 Challenges in Smart Grids and Energy Systems: Smart devices are increasingly integrated into smart grids and energy systems, where they play a crucial role in energy management and distribution. Cyberattacks on these devices can disrupt the balance and operation of the entire energy system, leading to inefficiencies, potential blackouts, and compromised energy security. Addressing the energy consumption of smart devices during cyberattacks is therefore vital for ensuring the stability and reliability of smart grids.
Detection of Energy Consumption Cyber Attacks on Smart Devices
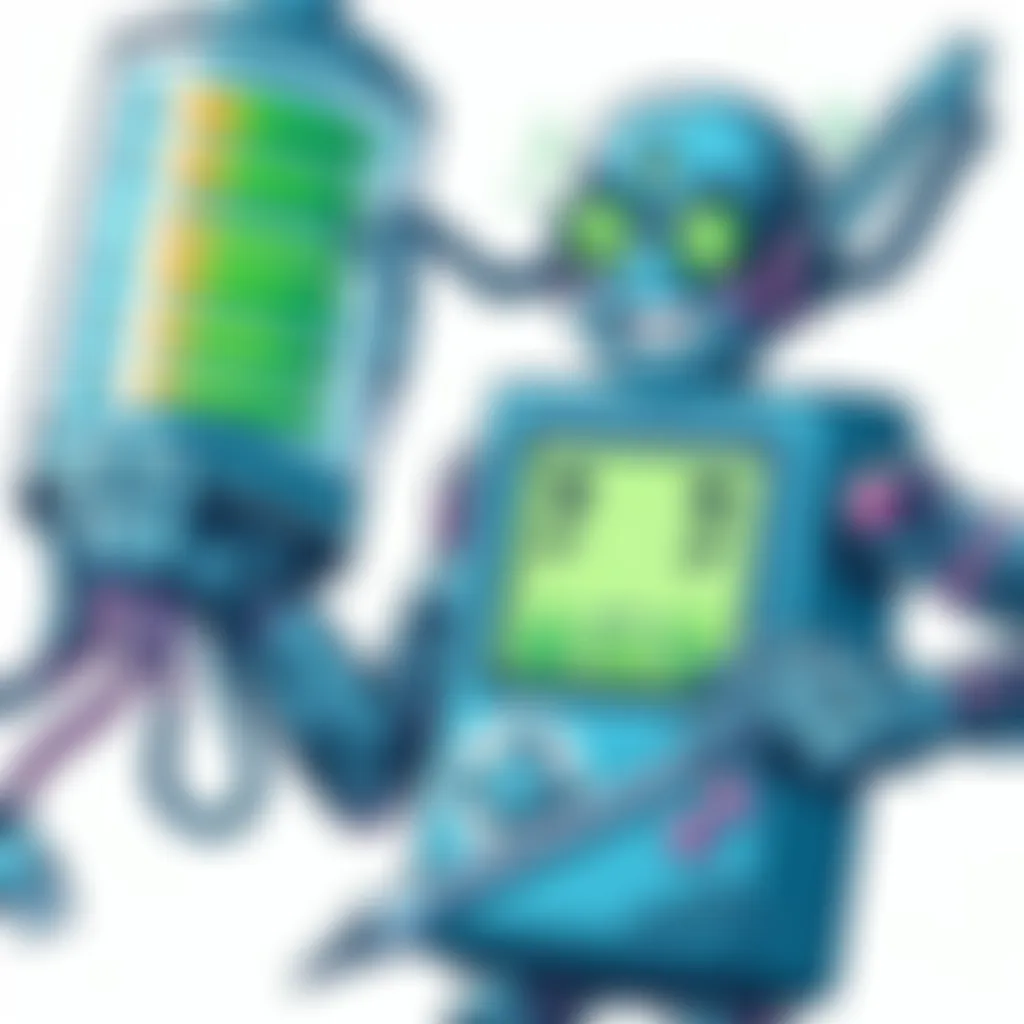
In a world where smart devices are supposed to make our lives easier, «Detection of Energy Consumption Cyber Attacks on Smart Devices» dives into the thrilling saga of how these gadgets can be turned against us. Imagine your smart fridge plotting is going to drain your energy bill while you sleep, or your thermostat conspiring with your toaster to launch a cyberattack. This paper heroically proposes a lightweight detection framework to save us from these nefarious appliances by analyzing their energy consumption patterns. Because, clearly, the best way to outsmart a smart device is to monitor how much juice it’s guzzling. So, next time your smart light bulb flickers, don’t worry—it’s just the algorithm doing its job.
---
The paper emphasizes the rapid integration of IoT technology into smart homes, highlighting the associated security challenges due to resource constraints and unreliable networks.
📌 Energy Efficiency: it emphasizes the significance of energy efficiency in IoT systems, particularly in smart home environments for comfort, convenience, and security.
📌 Vulnerability: it discusses the vulnerability of IoT devices to cyberattacks and physical attacks due to their resource constraints. It underscores the necessity of securing these devices to ensure their effective deployment in real-world scenarios.
📌 Proposed Detection Framework: The authors propose a detection framework based on analyzing the energy consumption of smart devices. This framework aims to classify the attack status of monitored devices by examining their energy consumption patterns.
📌 Two-Stage Approach: The methodology involves a two-stage approach. The first stage uses a short time window for rough attack detection, while the second stage involves more detailed analysis.
📌 Lightweight Algorithm: The paper introduces a lightweight algorithm designed to detect energy consumption attacks on smart home devices. This algorithm is tailored to the limited resources of IoT devices and considers three different protocols: TCP, UDP, and MQTT.
📌 Packet Reception Rate Analysis: The detection technique relies on analyzing the packet reception rate of smart devices to identify abnormal behavior indicative of energy consumption attacks.
Benefits
📌 Lightweight Detection Algorithm: The proposed algorithm is designed to be lightweight, making it suitable for resource constrained IoT devices. This ensures that the detection mechanism does not overly burden the devices it aims to protect.
📌 Protocol Versatility: The algorithm considers multiple communication protocols (TCP, UDP, MQTT), enhancing its applicability across various types of smart devices and network configurations.
📌 Two-Stage Detection Approach: The use of a two-stage detection approach (short and long-time windows) improves the accuracy of detecting energy consumption attacks while minimizing false positives. This method allows for both quick initial detection and detailed analysis.
📌 Real-Time Alerts: The framework promptly alerts administrators upon detecting an attack, enabling quick response and mitigation of potential threats.
📌 Effective Anomaly Detection: By measuring packet reception rates and analyzing energy consumption patterns, the algorithm effectively identifies deviations from normal behavior, which are indicative of cyberattacks.
Drawbacks
📌 Limited Attack Scenarios: The experimental setup has tested only specific types of attacks, which limit the generalizability of the results to other potential attack vectors not covered in the study.
📌 Scalability Concerns: While the algorithm is designed to be lightweight, its scalability in larger, more complex smart home environments with numerous devices and varied network conditions may require further validation.
📌 Dependency on Baseline Data: The effectiveness of the detection mechanism relies on accurate baseline measurements of packet reception rates and energy consumption. Any changes in the normal operating conditions of the devices could affect the baseline, potentially leading to false positives or negatives.
📌 Resource Constraints: Despite being lightweight, the algorithm still requires computational resources, which might be a challenge for extremely resource-limited devices. Continuous monitoring and analysis could also impact the battery life and performance of these devices.
Unpacking in more detail