Hacking the Hippocratic Oath. Forensic Fun with Medical IoT
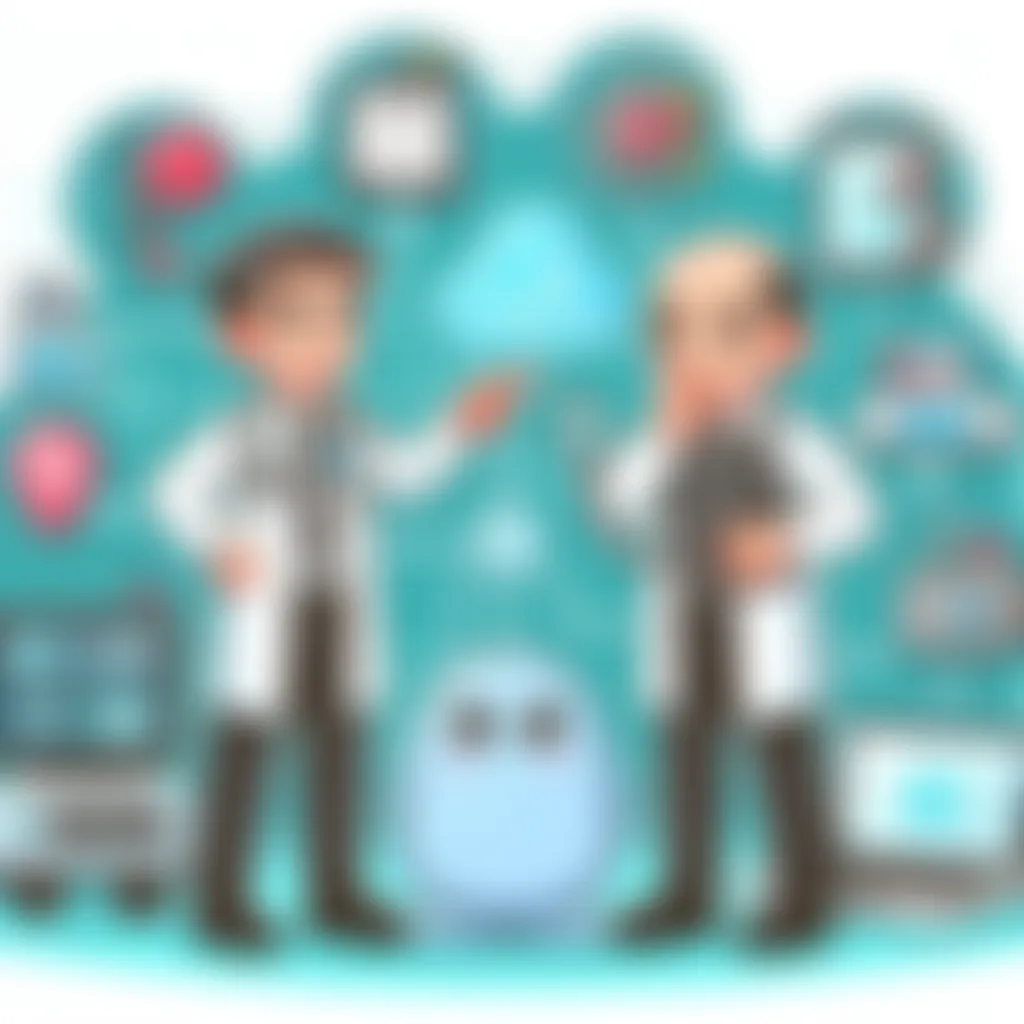
Hacking the Hippocratic Oath. Forensic Fun with Medical IoT [announcement]
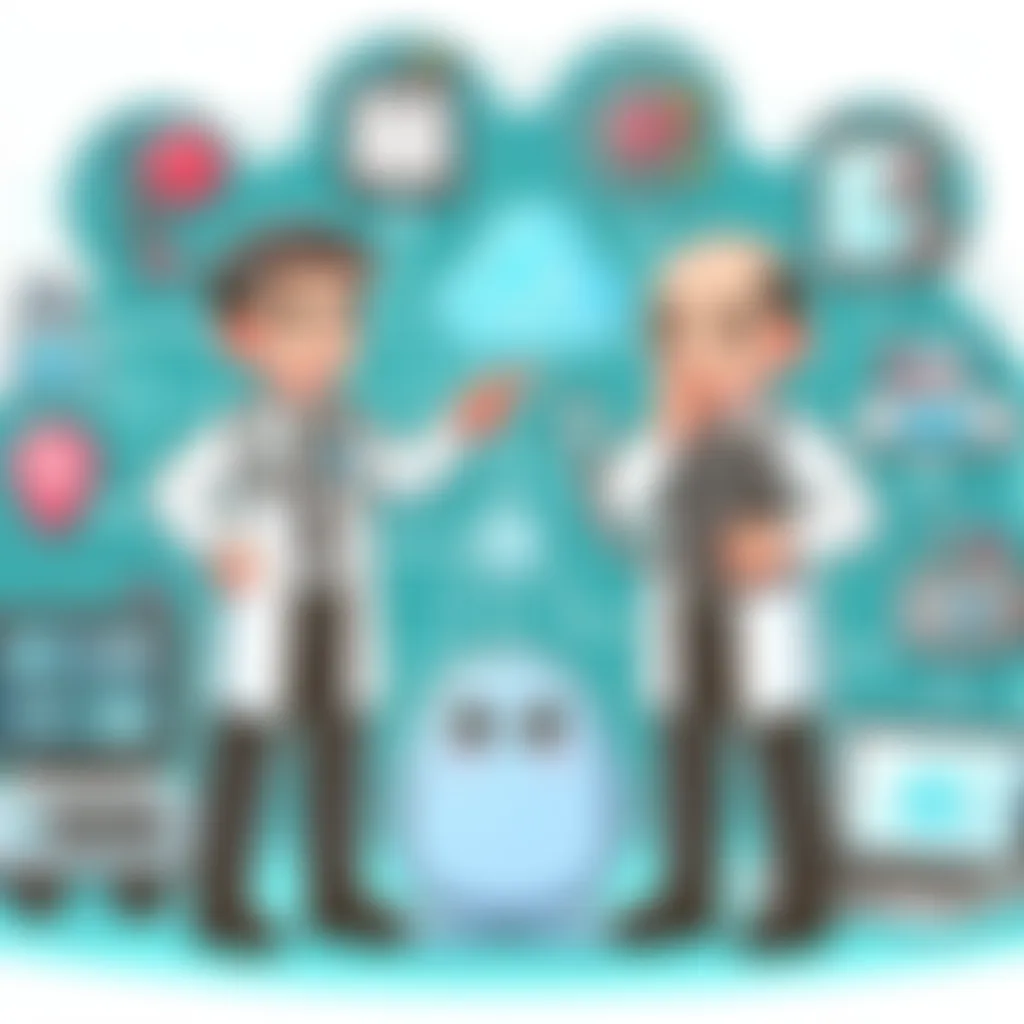
this document provides a comprehensive analysis of Medical Internet of Things (IoMT) Forensics, focusing on various critical aspects relevant to the field, including examination of current forensic methodologies tailored for IoT environments, highlighting their adaptability and effectiveness in medical contexts; techniques for acquiring digital evidence from medical IoT devices, considering the unique challenges posed by these devices; exploration of privacy issues and security vulnerabilities inherent in medical IoT systems, and how these impact forensic investigations; review of the tools and technologies used in IoT forensics, with a focus on those applicable to medical devices; analysis of real-world case studies where medical IoT devices played a crucial role in forensic investigations, providing practical insights and lessons learned.
This document offers a high-quality synthesis of the current state of Medical IoT Forensics, making it a valuable resource for security professionals, forensic investigators, and specialists across various industries. The insights provided can help enhance the understanding and implementation of effective forensic practices in the rapidly evolving landscape of medical IoT.
----
The rapid adoption of the Internet of Things (IoT) in the healthcare industry, known as the Internet of Medical Things (IoMT), has revolutionized patient care and medical operations. IoMT devices, such as wearable health monitors, implantable medical devices, and smart hospital equipment, generate and transmit vast amounts of sensitive data over networks.
Medical IoT network forensics is an emerging field that focuses on the identification, acquisition, analysis, and preservation of digital evidence from IoMT devices and networks. It plays a crucial role in investigating security incidents, data breaches, and cyber-attacks targeting healthcare organizations. The unique nature of IoMT systems, with their diverse range of devices, communication protocols, and data formats, presents significant challenges for traditional digital forensics techniques.
The primary objectives of medical IoT network forensics are:
📌 Incident Response: Rapidly respond to security incidents by identifying the source, scope, and impact of the attack, and gathering evidence to support legal proceedings or regulatory compliance.
📌 Evidence Acquisition: Develop specialized techniques to acquire and preserve digital evidence from IoMT devices, networks, and cloud-based systems while maintaining data integrity and chain of custody.
📌 Data Analysis: Analyze the collected data, including network traffic, device logs, and sensor readings, to reconstruct the events leading to the incident and identify potential vulnerabilities or attack vectors.
📌 Threat Intelligence: Leverage the insights gained from forensic investigations to enhance threat intelligence, improve security measures, and prevent future attacks on IoMT systems.
Medical IoT network forensics requires a multidisciplinary approach, combining expertise in digital forensics, cybersecurity, healthcare regulations, and IoT technologies. Forensic investigators must navigate the complexities of IoMT systems, including device heterogeneity, resource constraints, proprietary protocols, and the need to maintain patient privacy and data confidentiality.
MediHunt
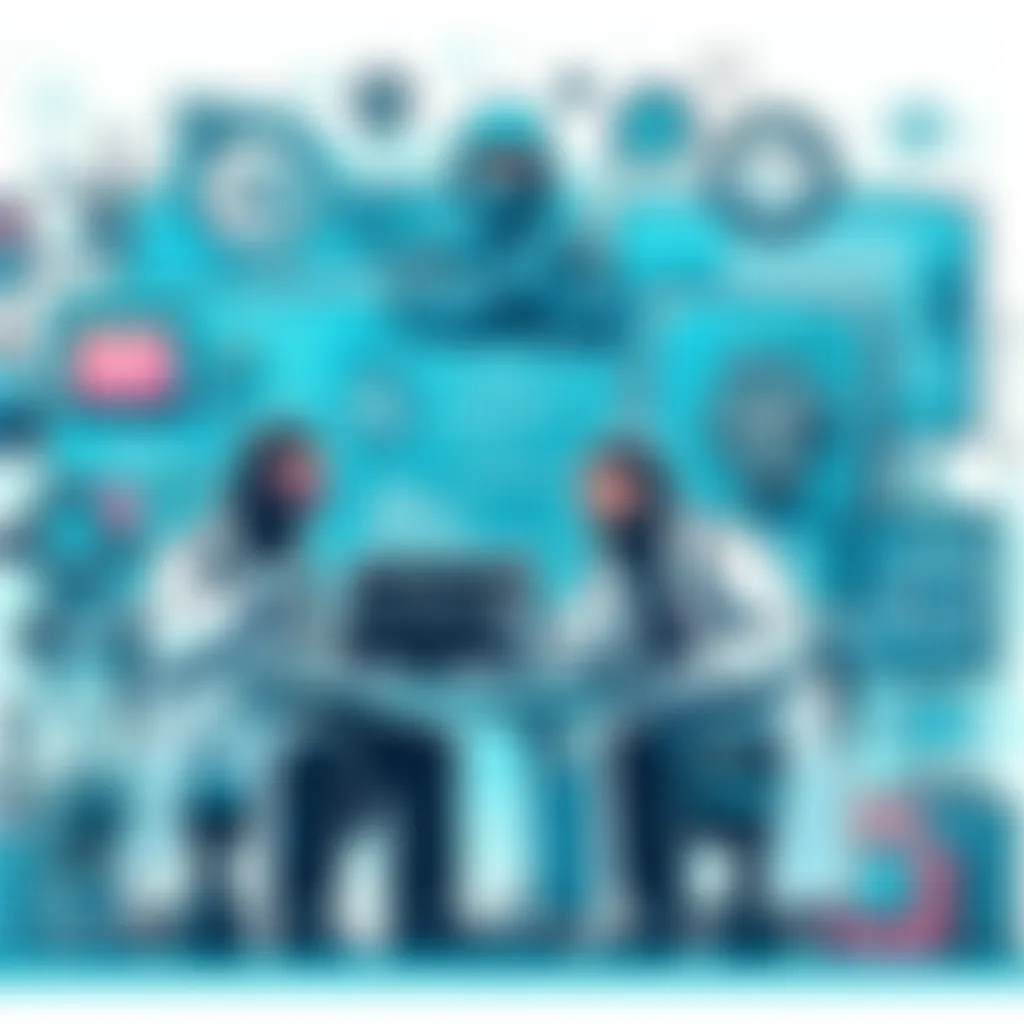
The paper «MediHunt: A Network Forensics Framework for Medical IoT Devices» is a real page-turner. It starts by addressing the oh-so-urgent need for robust network forensics in Medical Internet of Things (MIoT) environments. You know, those environments where MQTT (Message Queuing Telemetry Transport) networks are the darling of smart hospitals because of their lightweight communication protocol.
MediHunt is an automatic network forensics framework designed for real-time detection of network flow-based traffic attacks in MQTT networks. It leverages machine learning models to enhance detection capabilities and is suitable for deployment on those ever-so-resource-constrained MIoT devices. Because, naturally, that’s what we’ve all been losing sleep over.
These points set the stage for the detailed discussion of the framework, its experimental setup, and evaluation presented in the subsequent sections of the paper. Can’t wait to dive into those thrilling details!
---
The paper addresses the need for robust network forensics in Medical Internet of Things (MIoT) environments, particularly focusing on MQTT (Message Queuing Telemetry Transport) networks. These networks are commonly used in smart hospital environments for their lightweight communication protocol. It highlights the challenges in securing MIoT devices, which are often resource-constrained and have limited computational power. The lack of publicly available flow-based MQTT-specific datasets for training attack detection systems is mentioned as a significant challenge.
The paper presents MediHunt as an automatic network forensics solution designed for real-time detection of network flow-based traffic attacks in MQTT networks. It aims to provide a comprehensive solution for data collection, analysis, attack detection, presentation, and preservation of evidence. It is designed to detect a variety of TCP/IP layers and application layer attacks on MQTT networks. It leverages machine learning models to enhance the detection capabilities and is suitable for deployment on resource constrained MIoT devices.
The primary objective of the MediHunt is to strengthen the forensic analysis capabilities in MIoT environments, ensuring that malicious activities can be traced and mitigated effectively.
Benefits
📌 Real-time Attack Detection: MediHunt is designed to detect network flow-based traffic attacks in real-time, which is crucial for mitigating potential damage and ensuring the security of MIoT environments.
📌 Comprehensive Forensic Capabilities: The framework provides a complete solution for data collection, analysis, attack detection, presentation, and preservation of evidence. This makes it a robust tool for network forensics in MIoT environments.
📌 Machine Learning Integration: By leveraging machine learning models, MediHunt enhances its detection capabilities. The use of a custom dataset that includes flow data for both TCP/IP layer and application layer attacks allows for more accurate and effective detection of a wide range of cyber-attacks.
📌 High Performance: The framework has demonstrated high performance, with F1 scores and detection accuracy exceeding 0.99 and indicates that it is highly reliable in detecting attacks on MQTT networks.
📌 Resource Efficiency: Despite its comprehensive capabilities, MediHunt is designed to be resource-efficient, making it suitable for deployment on resource-constrained MIoT devices like Raspberry Pi.
Drawbacks
📌 Dataset Limitations: While MediHunt uses a custom dataset for training its machine learning models, the creation and maintenance of such datasets can be challenging. The dataset needs to be regularly updated to cover new and emerging attack scenarios.
📌 Resource Constraints: Although MediHunt is designed to be resource-efficient, the inherent limitations of MIoT devices, such as limited computational power and memory, can still pose challenges. Ensuring that the framework runs smoothly on these devices without impacting their primary functions can be difficult.
📌 Complexity of Implementation: Implementing and maintaining a machine learning-based network forensics framework can be complex. It requires expertise in cybersecurity and machine learning, which may not be readily available in all healthcare settings.
📌 Dependence on Machine Learning Models: The effectiveness of MediHunt heavily relies on the accuracy and robustness of its machine learning models. These models need to be trained on high-quality data and regularly updated to remain effective against new types of attacks.
📌 Scalability Issues: While the framework is suitable for small-scale deployments on devices like Raspberry Pi, scaling it up to larger, more complex MIoT environments may present additional challenges. Ensuring consistent performance and reliability across a larger network of devices can be difficult
Unpacking in more detail
Взлом клятвы Гиппократа. Криминалистическая забава с медицинским Интернетом вещей
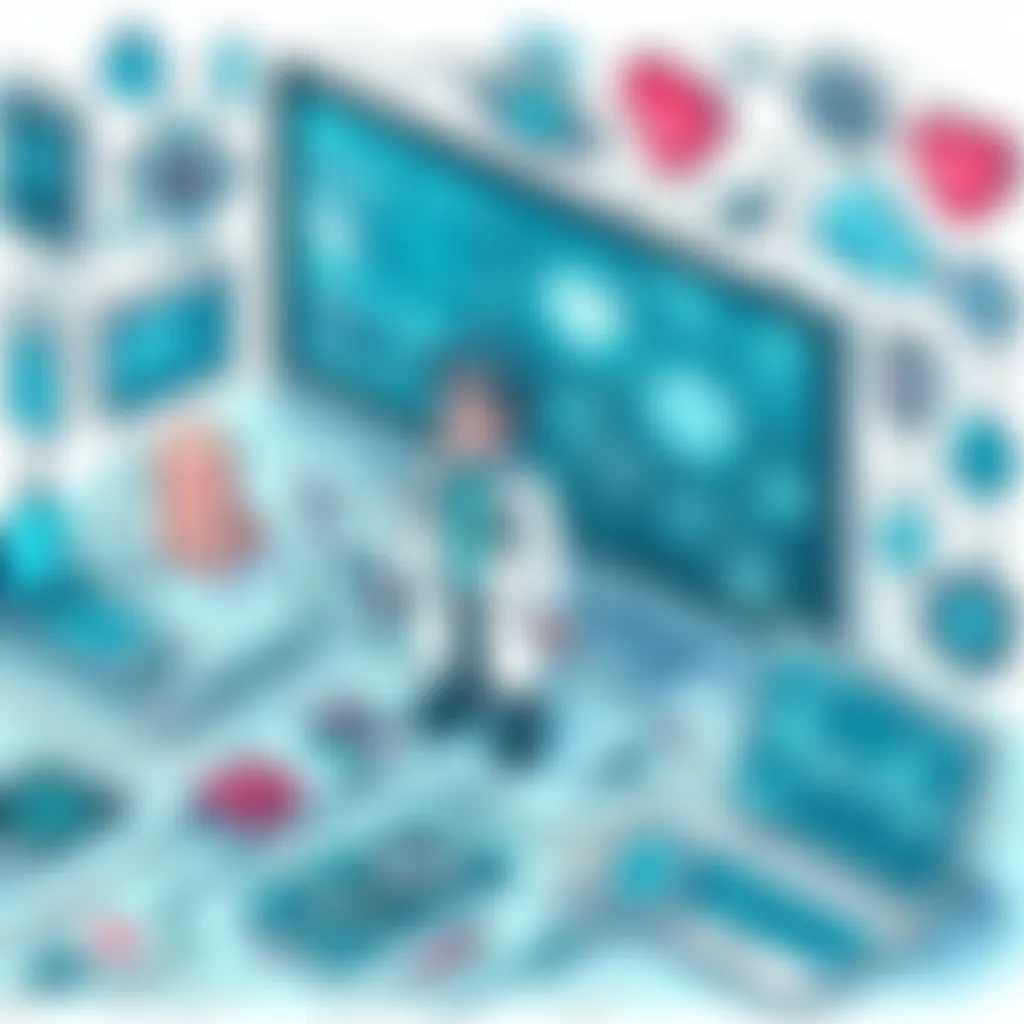
Взлом клятвы Гиппократа. Криминалистическая забава с медицинским Интернетом вещей. Анонс
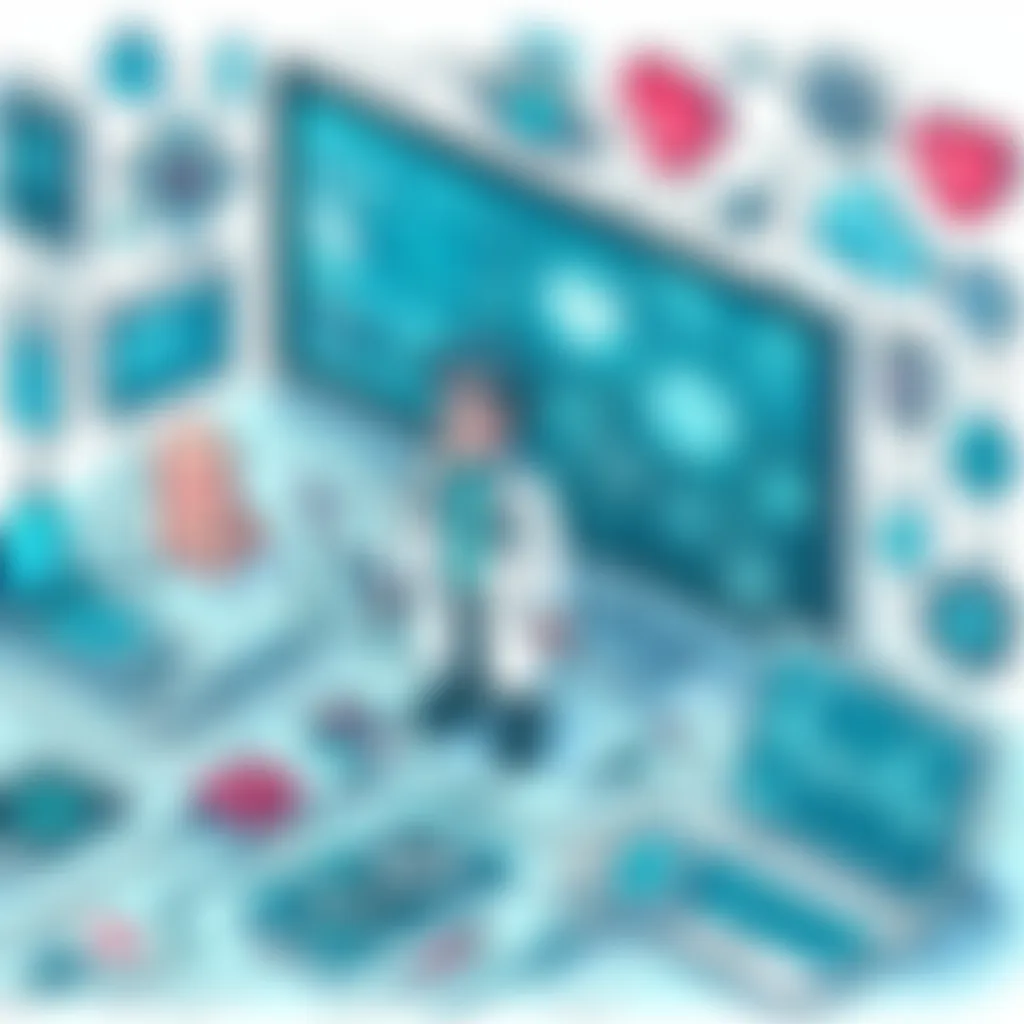
в документе содержится криминалистический анализ области медицинского интернета вещей (IoMT), различных критических аспектов, имеющим отношение к данной области, включая разработку современных и эффективных методик forensics-анализа; методов получения данных с медицинских устройств; изучение проблем конфиденциальности и безопасности медицинских систем, и того, как это влияет на forensics-исследования; обзор forensics-технологий, с акцентом применимые к медицинским устройствам; анализ примеров из реальной практики.
Этот документ предлагает качественный материал текущего состояния медицинской криминалистики, что делает его ценным ресурсом для специалистов в области безопасности, forensics-специалистов и специалистов из различных отраслей промышленности. Представленная информация может помочь улучшить понимание и внедрение эффективных методов forensics-анализа.
-------
Быстрое внедрение Интернета вещей (IoT) в отрасли здравоохранения, известного как Интернет медицинских вещей (IoMT), произвело революцию в уходе за пациентами и медицинских операциях. Устройства IoMT, такие как имплантируемые медицинские устройства, носимые медицинские мониторы и интеллектуальное больничное оборудование, формируют и передают огромные объёмы конфиденциальных данных по сетям.
Криминалистика медицинских сетей Интернета вещей — это развивающаяся область, которая фокусируется на идентификации, сборе, анализе и сохранении цифровых доказательств с устройств и сетей IoMT. Она играет решающую роль в расследовании инцидентов безопасности, утечек данных и кибератак, направленных против организаций здравоохранения. Уникальная природа систем IoMT с их разнообразным набором устройств, протоколов связи и форматов данных создаёт значительные проблемы для традиционных методов цифровой криминалистики.
Основными задачами forensics-анализа медицинских сетей Интернета вещей являются:
📌 Реагирование на инциденты: Быстрое реагирование на инциденты безопасности путём определения источника, масштабов и последствий атаки, а также сбора доказательств или соблюдения нормативных требований.
📌 Сбор доказательств: Разработка специализированных методов получения и сохранения цифровых доказательств с устройств, сетей и облачных систем IoMT при сохранении целостности данных и цепочки хранения.
📌 Анализ данных: Анализ собранных данных, включая сетевой трафик, журналы устройств и показания датчиков для реконструкции событий, приведшие к инциденту, и определить потенциальные уязвимости или векторы атак.
📌 Анализ угроз: использование информации, полученной в ходе исследований для улучшения анализа угроз, совершенствования мер безопасности и предотвращения атак на IoMT.
Криминалистика медицинских сетей Интернета вещей требует междисциплинарного подхода, сочетающего опыт цифровой forensics-анализа, кибербезопасности, особенностей сектора здравоохранения и технологий Интернета вещей. Forensics-исследователи должны ориентироваться в сложностях систем IoMT, включая неоднородность устройств, ограниченность ресурсов, проприетарные протоколы и необходимость сохранения конфиденциальности пациентов и данных.
MediHunt
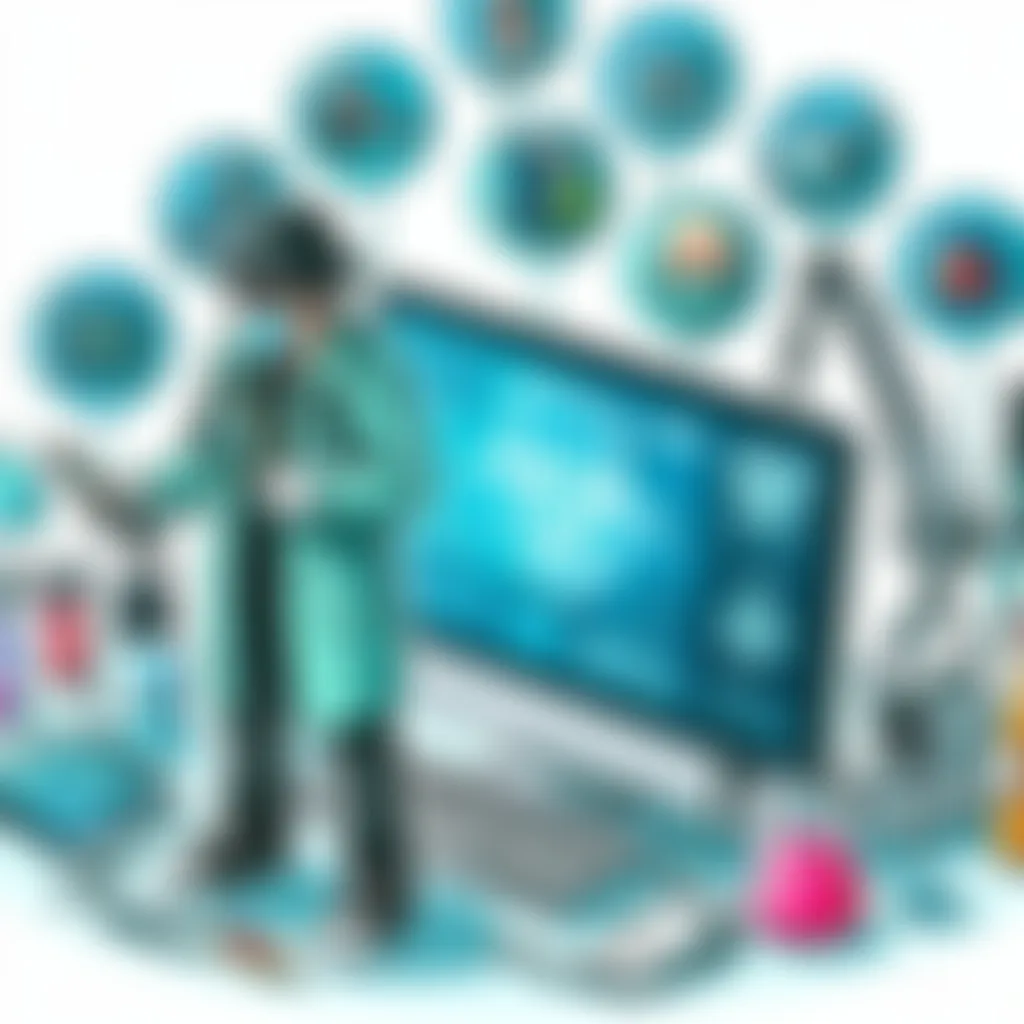
Статья " MediHunt: A Network Forensics Framework for Medical IoT Devices» — это настоящий прорыв. Она начинается с рассмотрения насущной потребности в надёжной сетевой криминалистике в среде медицинского Интернета вещей (MIoT). Вы знаете, что среды, в которых используются сети передачи телеметрии с использованием MQTT (Message Queuing Telemetry Transport), являются любимыми для умных больниц из-за их облегчённого протокола связи.
MediHunt — это платформа автоматической сетевой криминалистики, предназначенная для обнаружения атак на сетевой трафик в сетях MQTT в режиме реального времени. Она использует модели машинного обучения для расширения возможностей обнаружения и подходит для развёртывания на устройствах MIoT с ограниченными ресурсами. Потому что, естественно, именно из-за этого мы все потеряли сон.
Эти аспекты — отличная почва для подробного обсуждения фреймворка, его экспериментальной установки и оценки. Вам уже не терпится погрузиться в эти захватывающие подробности?
-------
В документе рассматривается необходимость надёжной сетевой криминалистики в медицинских средах Интернета вещей (MIoT), особенно с упором на сети MQTT. Эти сети обычно используются в интеллектуальных больничных средах благодаря их облегчённому протоколу связи. Освещаются проблемы обеспечения безопасности устройств MIoT, которые часто ограничены в ресурсах и обладают ограниченной вычислительной мощностью. В качестве серьёзной проблемы упоминается отсутствие общедоступных потоковых наборов данных, специфичных для MQTT, для обучения систем обнаружения атак.
MediHunt как решение для автоматизированной сетевой криминалистики, предназначенное для обнаружения атак на основе сетевого трафика в сетях MQTT в режиме реального времени. Его цель — предоставить комплексное решение для сбора данных, анализа, обнаружения атак, представления и сохранения доказательств. Он разработан для обнаружения различных уровней TCP / IP и атак прикладного уровня в сетях MQTT и использует модели машинного обучения для расширения возможностей обнаружения и подходит для развёртывания на устройствах MIoT с ограниченными ресурсами.
Преимущества
📌 Обнаружение атак в режиме реального времени: MediHunt предназначен для обнаружения атак на основе сетевого трафика в режиме реального времени для уменьшения потенциального ущерба и обеспечения безопасности сред MIoT.
📌 Комплексные возможности криминалистики: Платформа предоставляет комплексное решение для сбора данных, анализа, обнаружения атак, представления и сохранения доказательств. Это делает его надёжным инструментом сетевой криминалистики в средах MIoT.
📌 Интеграция с машинным обучением: Используя модели машинного обучения, MediHunt расширяет свои возможности обнаружения. Использование пользовательского набора данных, который включает данные о потоках как для атак уровня TCP/IP, так и для атак прикладного уровня, позволяет более точно и эффективно обнаруживать широкий спектр кибератак.
📌 Высокая производительность: решение показало высокую производительность, получив баллы F1 и точность обнаружения, превышающую 0,99 и указывает на то, что она обладает высокой надёжностью при обнаружении атак на сети MQTT.
📌 Эффективность использования ресурсов: несмотря на свои широкие возможности, MediHunt разработан с учётом экономии ресурсов, что делает его подходящим для развёртывания на устройствах MIoT с ограниченными ресурсами (raspberry Pi).
Недостатки
📌 Ограничения набора данных: хотя MediHunt использует пользовательский набор данных для обучения своих моделей машинного обучения, создание и обслуживание таких наборов данных может быть сложной задачей. Набор данных необходимо регулярно обновлять, чтобы охватывать новые и зарождающиеся сценарии атак.
📌 Ограничения ресурсов: хотя MediHunt разработан с учётом экономии ресурсов, ограничения, присущие устройствам MIoT, такие как ограниченная вычислительная мощность и память, все ещё могут создавать проблемы. Обеспечить бесперебойную работу фреймворка на этих устройствах без ущерба для их основных функций может быть непросто.
📌 Сложность реализации: Внедрение и поддержка платформы сетевой криминалистики на основе машинного обучения может быть сложной задачей. Это требует опыта в области кибербезопасности и машинного обучения, который может быть доступен не во всех медицинских учреждениях.
📌 Зависимость от моделей машинного обучения: Эффективность MediHunt в значительной степени зависит от точности и надёжности его моделей машинного обучения. Эти модели необходимо обучать на высококачественных данных и регулярно обновлять, чтобы они оставались эффективными против новых типов атак.
📌 Проблемы с масштабируемостью: хотя платформа подходит для небольших развёртываний на устройствах типа Raspberry Pi, ее масштабирование до более крупных и сложных сред MIoT может вызвать дополнительные проблемы. Обеспечение стабильной производительности и надёжности в более крупной сети устройств может быть затруднено
Подробный разбор
Инфобез в медицине
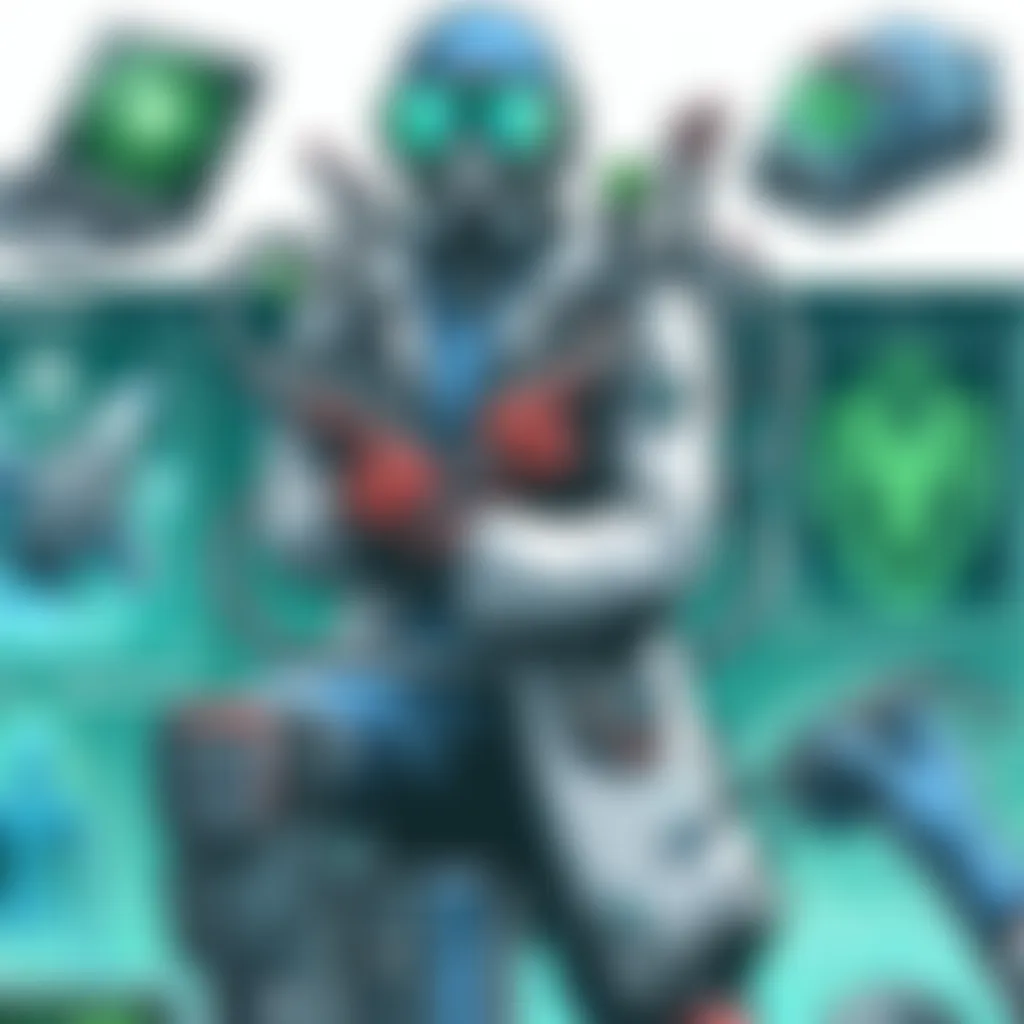
Давайте оценим чудеса интеграции устройств Интернета вещей (IoT) в здравоохранение. Что может пойти не так с подключением всех мыслимых медицинских устройств к Интернету? Кардиостимуляторы, аппараты магнитно-резонансной томографии, умные инфузионные насосы — все устройства просят: «Взломайте нас, пожалуйста!»
Погружаясь в пучину угроз кибербезопасности, не будем забывать о том, как замечательно, что ритм вашего сердца зависит от чего-то такого стабильного и безопасного, как Интернет. И кто мог бы не порадоваться тому, что ваши медицинские данные хранятся в облаке и вот-вот станут достоянием общественности? Соблюдение индустриальных требований и практик волшебным образом предотвратят все киберугрозы. Потому что хакеры полностью соблюдают правила, и их определённо отпугивают «лучшие намерения» медицинской организации.
Последствия кибератаки на медицинские технологии сказываются не только на поставщиках медицинских услуг, но и на страховых компаниях, фармацевтических компаниях и даже службах неотложной помощи. В больницах царит хаос, лечение откладывается, а безопасность пациентов находится под угрозой — это идеальный вариант. Но давайте не будем забывать и о невоспетых героях: компаниях, занимающихся кибербезопасностью, которые радостно потирают руки, когда спрос на их услуги стремительно растёт.
Добро пожаловать в будущее здравоохранения, где ваше медицинское устройство может стать частью очередной крупной утечки данных. Спите спокойно!
-----
В этом документе освещаются киберугрозы медицинским и коммуникационным технологиям и потенциальные риски и уязвимости в связанных протоколах. Документ разработан для того, чтобы помочь организациям здравоохранения и медицинским работникам понять важность обеспечения безопасности их технологических систем для защиты данных пациентов и обеспечения непрерывности оказания медицинской помощи.
Подробный разбор
Medical Security
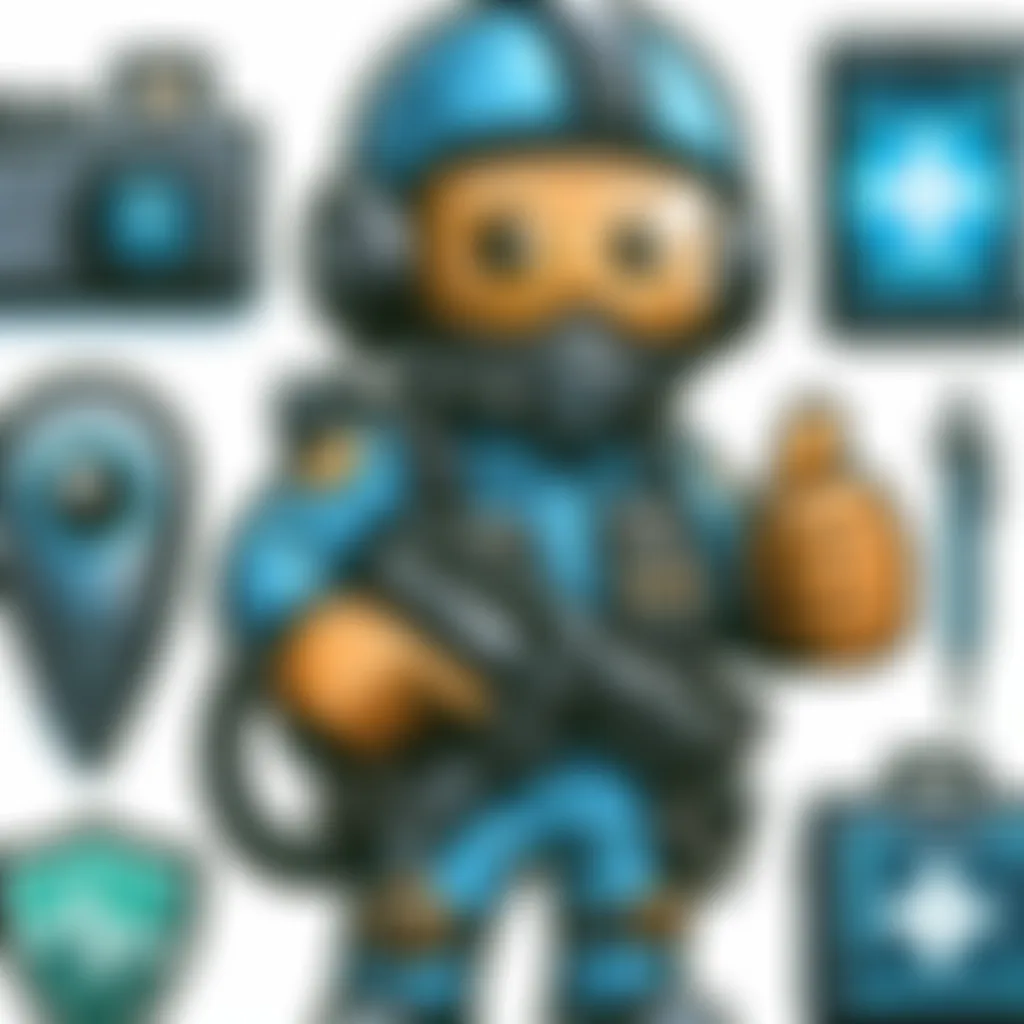
Let’s all take a moment to appreciate the marvels of integrating Internet of Things (IoT) devices into healthcare. What could possibly go wrong with connecting every conceivable medical device to the internet? Pacemakers, MRI machines, smart infusion pumps — it’s like every device is screaming, «Hack me, please!»
As we dive into the abyss of cybersecurity threats, let’s not forget the sheer brilliance of having your heart’s pacing dependent on something as stable and secure as the internet. And who could overlook the excitement of having your medical data floating around in the cloud, just a breach away from becoming public knowledge? But wait, there’s more! Compliance with HIPAA and adherence to best practices will magically ward off all cyber threats. Because hackers totally play by the rules and are definitely deterred by a healthcare organization’s best intentions.
The ripple effects of a cyber-attack on medical technology affect not just healthcare providers but also dragging down insurance companies, pharmaceuticals, and even emergency services into the mire. Hospitals in chaos, treatments delayed, and patient safety compromised — it’s the perfect storm. But let’s not forget the unsung heroes: cybersecurity firms, rubbing their hands in glee as the demand for their services skyrockets.
Welcome to the future of healthcare, where your medical device might just be part of the next big data breach headline. Sleep tight!
-----
This document highlights the cyber threats to medical technology and communication technology protocols and outlines the potential risks and vulnerabilities in these systems. It is designed to help healthcare organizations and medical professionals understand the importance of securing their technology systems to protect patient data and ensure the continuity of care.
Unpacking in more detail