MediHunt
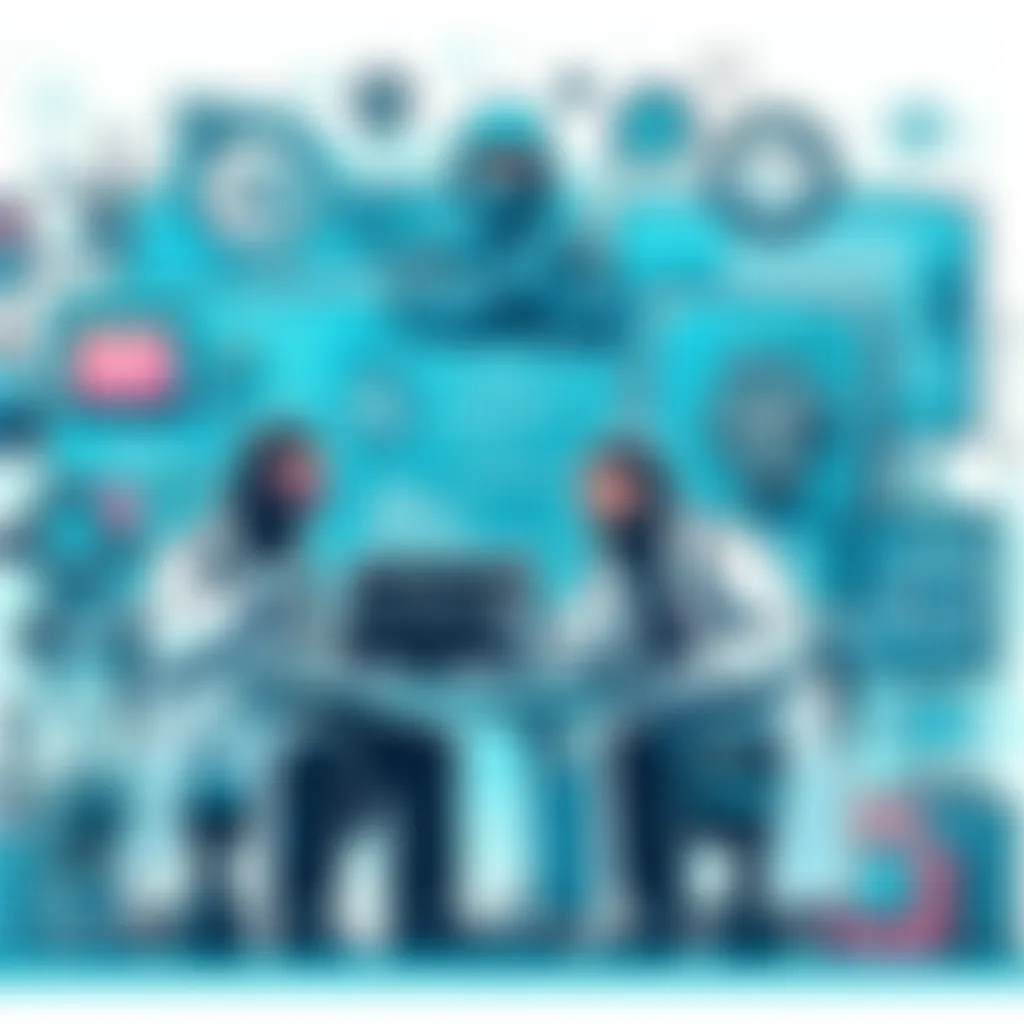
The paper «MediHunt: A Network Forensics Framework for Medical IoT Devices» is a real page-turner. It starts by addressing the oh-so-urgent need for robust network forensics in Medical Internet of Things (MIoT) environments. You know, those environments where MQTT (Message Queuing Telemetry Transport) networks are the darling of smart hospitals because of their lightweight communication protocol.
MediHunt is an automatic network forensics framework designed for real-time detection of network flow-based traffic attacks in MQTT networks. It leverages machine learning models to enhance detection capabilities and is suitable for deployment on those ever-so-resource-constrained MIoT devices. Because, naturally, that’s what we’ve all been losing sleep over.
These points set the stage for the detailed discussion of the framework, its experimental setup, and evaluation presented in the subsequent sections of the paper. Can’t wait to dive into those thrilling details!
---
The paper addresses the need for robust network forensics in Medical Internet of Things (MIoT) environments, particularly focusing on MQTT (Message Queuing Telemetry Transport) networks. These networks are commonly used in smart hospital environments for their lightweight communication protocol. It highlights the challenges in securing MIoT devices, which are often resource-constrained and have limited computational power. The lack of publicly available flow-based MQTT-specific datasets for training attack detection systems is mentioned as a significant challenge.
The paper presents MediHunt as an automatic network forensics solution designed for real-time detection of network flow-based traffic attacks in MQTT networks. It aims to provide a comprehensive solution for data collection, analysis, attack detection, presentation, and preservation of evidence. It is designed to detect a variety of TCP/IP layers and application layer attacks on MQTT networks. It leverages machine learning models to enhance the detection capabilities and is suitable for deployment on resource constrained MIoT devices.
The primary objective of the MediHunt is to strengthen the forensic analysis capabilities in MIoT environments, ensuring that malicious activities can be traced and mitigated effectively.
Benefits
📌 Real-time Attack Detection: MediHunt is designed to detect network flow-based traffic attacks in real-time, which is crucial for mitigating potential damage and ensuring the security of MIoT environments.
📌 Comprehensive Forensic Capabilities: The framework provides a complete solution for data collection, analysis, attack detection, presentation, and preservation of evidence. This makes it a robust tool for network forensics in MIoT environments.
📌 Machine Learning Integration: By leveraging machine learning models, MediHunt enhances its detection capabilities. The use of a custom dataset that includes flow data for both TCP/IP layer and application layer attacks allows for more accurate and effective detection of a wide range of cyber-attacks.
📌 High Performance: The framework has demonstrated high performance, with F1 scores and detection accuracy exceeding 0.99 and indicates that it is highly reliable in detecting attacks on MQTT networks.
📌 Resource Efficiency: Despite its comprehensive capabilities, MediHunt is designed to be resource-efficient, making it suitable for deployment on resource-constrained MIoT devices like Raspberry Pi.
Drawbacks
📌 Dataset Limitations: While MediHunt uses a custom dataset for training its machine learning models, the creation and maintenance of such datasets can be challenging. The dataset needs to be regularly updated to cover new and emerging attack scenarios.
📌 Resource Constraints: Although MediHunt is designed to be resource-efficient, the inherent limitations of MIoT devices, such as limited computational power and memory, can still pose challenges. Ensuring that the framework runs smoothly on these devices without impacting their primary functions can be difficult.
📌 Complexity of Implementation: Implementing and maintaining a machine learning-based network forensics framework can be complex. It requires expertise in cybersecurity and machine learning, which may not be readily available in all healthcare settings.
📌 Dependence on Machine Learning Models: The effectiveness of MediHunt heavily relies on the accuracy and robustness of its machine learning models. These models need to be trained on high-quality data and regularly updated to remain effective against new types of attacks.
📌 Scalability Issues: While the framework is suitable for small-scale deployments on devices like Raspberry Pi, scaling it up to larger, more complex MIoT environments may present additional challenges. Ensuring consistent performance and reliability across a larger network of devices can be difficult
Unpacking in more detail